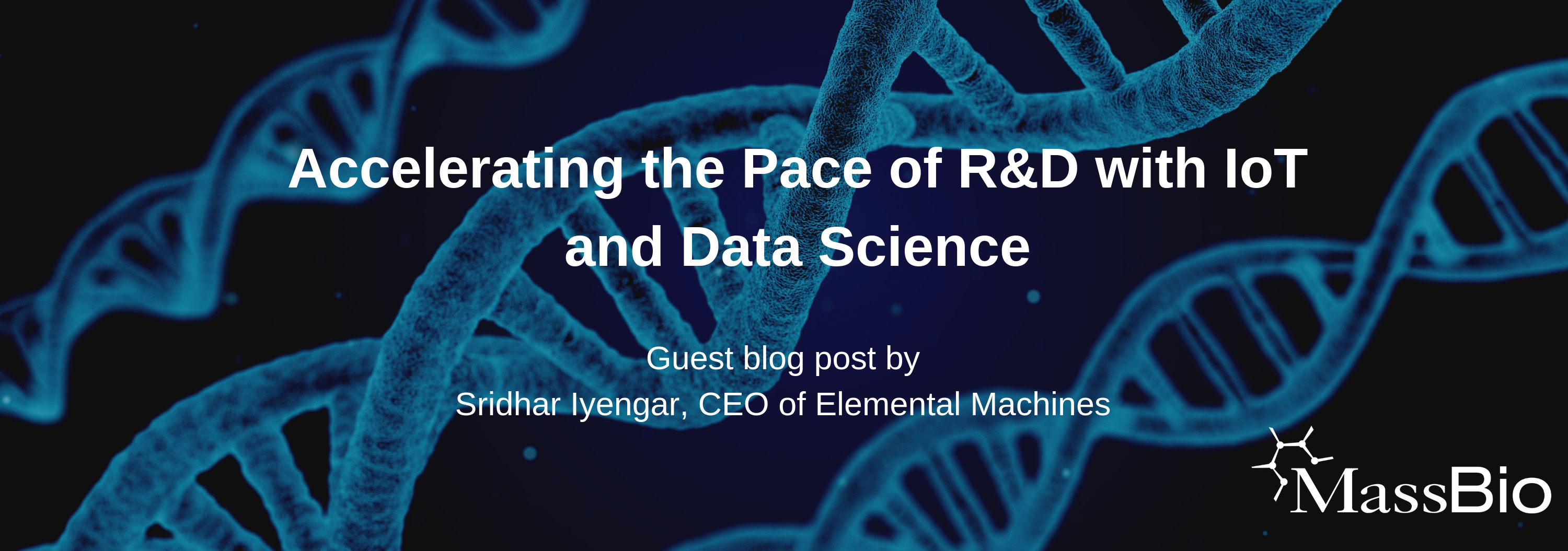
Over the last fifty years, R&D expenditures on new drug and biologics for FDA approval have grown exponentially, while the total number of new drug and biologics introduced onto the market has grown linearly. During this same time period, computing power has also grown exponentially, as patterned by Moore’s Law. How is this possible? With the advent of new technology, why hasn’t the life sciences industry been able to move at a faster rate to bring new therapies onto the market?
Let’s explore some possible reasons. The first explanation is FDA regulations. In the aftermath of the thalidomide disaster, the FDA tightened regulations for the sake of public health. Additionally, both the FDA and industry cautiously navigated regulatory pathways for new biotechnology-derived therapies in the late 1980s and early 1990s. Another explanation is that scientific research findings are not reproducible. In fact, studies have shown that 50% of findings are not reproducible. Common issues include variability in material storage, poor study design, and data analysis issues. The focus of this blog addresses the reproducibility problem.
So why are results from scientific experiments so difficult to reproduce? There are countless possibilities, but let’s look at two of of the biggest contributors. The first is the lack of automated data capture from mission-critical equipment. Current processes are manual without protections in place to preserve data integrity and promote regulatory compliance. The second contributor is the segregation of data into silos. In order to ‘debug’ experiments, scientists must correlate data and metadata from various sources, such as cold storage parameters, process parameters, etc. Today, these tend to be manual, tedious processes.
Wouldn’t it be great to have a way to measure everything and automatically make sense of it all? That’s what many companies envision when they think about a new emerging trend called the “Lab of the Future”.
The Lab of the Future is an IoT-equipped continuous data collection platform consisting of environmental sensors and add-on IoT-equipped hardware for legacy lab equipment. These tools send data to the cloud for secure data archival, real-time alerts, and remote monitoring. We are able to aggregate data from various sources and correlate experimental results to relevant inputs such as material storage, environmental parameters, time-dependencies, etc. The end results are accuracy, reproducibility, and optimization.
Figure 1: The foundation for the Lab of the Future hardware for continuous data collection and cloud connectivity to achieve optimization, accuracy, and repeatability
So what are some real-world examples of how life science companies have benefited from the Lab of the Future? In its simplest form, the Lab of the Future offers real-time updates to capture critical events that could lead to catastrophic failures. An example of this may be when an incubator growing a therapeutic cell line loses power due to a power outage.
While an outage like that may be easy to spot, a more challenging problem is spotting subtle changes whose effects may not be seen for weeks or months. Take for instance the following real-life examples:
- Several incubators within a laboratory were serviced and calibrated per company SOPs. During the service procedure, incubator settings, including setpoints, were reset to factory defaults. When the equipment was available for use, the lab manager was able to immediately identify the change in setpoints and correct them before any team member began incubation steps.
- Another team observed differences in cell growth from one of their shaker incubators. They ultimately realized that one of their shakers literally had a screw loose, causing a slightly different motion/vibration pattern, which in turn, affected the aeration of those cells. Now the team has added a motion sensor on each shaker to ensure that the set points match the actual motion. They value review real-time feedback for troubleshooting potential issues.
By equipping incubators with various IoT sensors and leveraging machine learning to spot anomalies, R&D teams can focus on their core competencies, i.e. developing novel therapeutics in this case, rather than troubleshooting mission-critical equipment.
The benefits and applications behind the Lab of the Future concept extend beyond the R&D phase. Manufacturing processes must also be ‘debugged’ to achieve reproducibility and optimize yield. After all, isn’t a manufacturing line essentially a lab-based protocol scaled up to higher volumes?
The deployment of IoT-enabled sensors allows for real-time correlation of intermediate processes with spatial, environmental, and instrument parameters. Manufacturing teams have used fleets of environmental sensors to uncover subtle but consequential temperature and humidity gradients across their plant floors.
Figure 2: Real-time equipment and environmental parameters overlay to identify sources of variability on the production floor.
One manufacturing team observed that raw materials processed on only one manufacturing line produced desirable finished product. The team adjusted environmental parameters on the underperforming manufacturing lines to remove gradients and repeatedly obtained desirable finished product from all lines, and they now have a system in place to ensure compliance moving forward.
The Life Sciences Industry can greatly benefit from Lab of the Future solutions that integrate IoT and Data Science. These tools can quickly and effectively surface unique (often hidden) patterns and events compared to traditional, manual methods to accelerate workflows, reduce time to market, and lower the cost of compliance to regulatory standards. Thus, the Lab of the Future is a welcome addition to the life sciences!